Audio-Morph GAN
Video games and movies often require custom sound effects. But these can be expensive and time consuming to record and produce. Therefore, we investigated how to generate environmental sounds with the ability to control various aspects of these sounds. For example, we would like to be able to generate wind sounds at low or high wind strength, or the sound of rain when it drizzles or buckets down.
We achieve this using a data-driven approach to control the process of synthesis of environmental sounds using Generative Adversarial Networks (GAN). We conditioned the GAN on “soft-labels” extracted from an audio classifier trained on a target set of audio texture classes. We demonstrate that interpolation between such conditions or control vectors provide smooth morphing between the generated audio textures, and show similar or better audio texture morphing capability compared to the state-of-the-art methods, while remaining consistent with the intended control parameters.
Audio examples generated from our model and code are available here: https://animatedsound.com/research/morphgan_icassp2023
This is a step towards a general data-driven approach to designing generative audio models with customized controls capable of traversing out-of-distribution regions for novel sound synthesis. Future work will include investigating unsupervised ways of inducing controllability in these models, and exploring controllability of other dimensions of environmental sounds such as emotions.
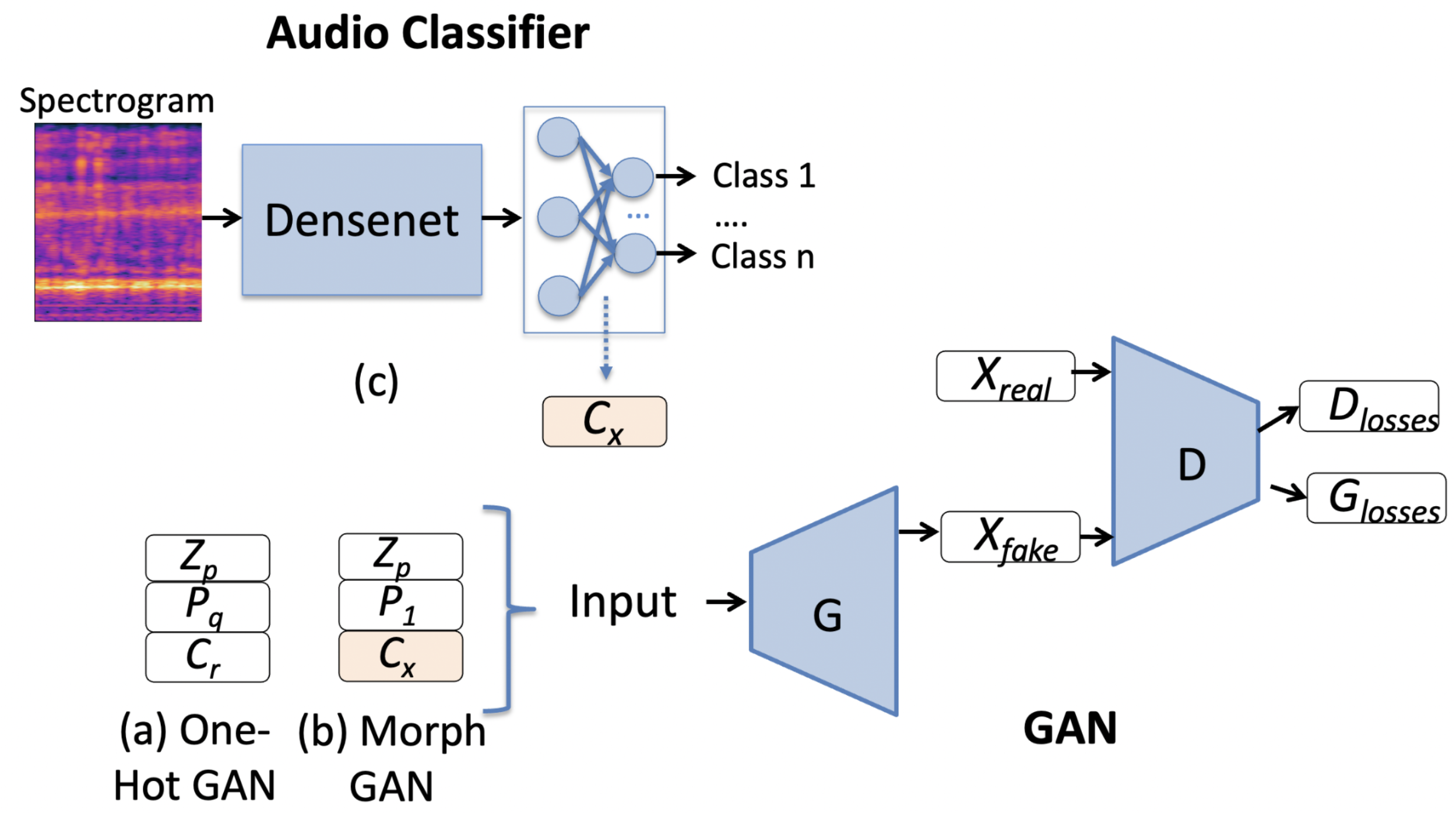
PUBLICATIONS
Towards Controllable Audio Texture Morphing
Gupta C*., Kamath P*., Wei. Y., Li, Z., Nanayakkara S.C., Wyse L. 2023. Towards Controllable Audio Texture Morphing. In 48th IEEE International Conference on Acoustics, Speech, and Signal Processing (ICASSP '23), 4-10 June, 2023, Rhodes Island, Greece.