Target Driven Navigation
Visual navigation is a core problem in robotics and machine vision. Previous research used map-based, map-building or map-less navigation strategies. The first two approaches were favored in the past, however, they essentially depend on the accurate mapping of the environment and a careful human-guided training phase, which overall limits generalizability. With recent developments in Deep Reinforcement Learning (DRL), map-less navigation experienced major advancements. A current challenge for DRL algorithms is learning new tasks or goals. This ability is called transfer learning. To cope with the challenges in transfer learning and performance, we present a novel approach, using Universal Successor Features (USF). We propose several models that we applied for the task of target driven visual navigation in a complex photo-realistic environment using a simulator named as AI2THOR. With the evaluation of our proposed models in AI2THOR, we demonstrate that an agent is able to improve the Transfer Reinforcement Learning ability.
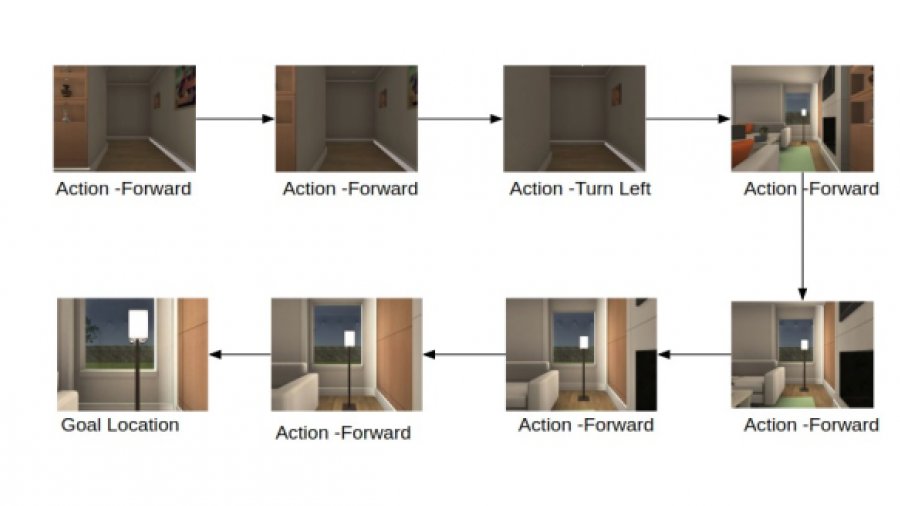
PUBLICATIONS
Target Driven Visual Navigation with Hybrid Asynchronous Universal Successor Representations
Siriwardhana, S., Weerasekera, R. and Nanayakkara, S.C, 2018, Target Driven Visual Navigation with Hybrid Asynchronous Universal Successor Representations. Deep RL Workshop NeurIPS 2018.